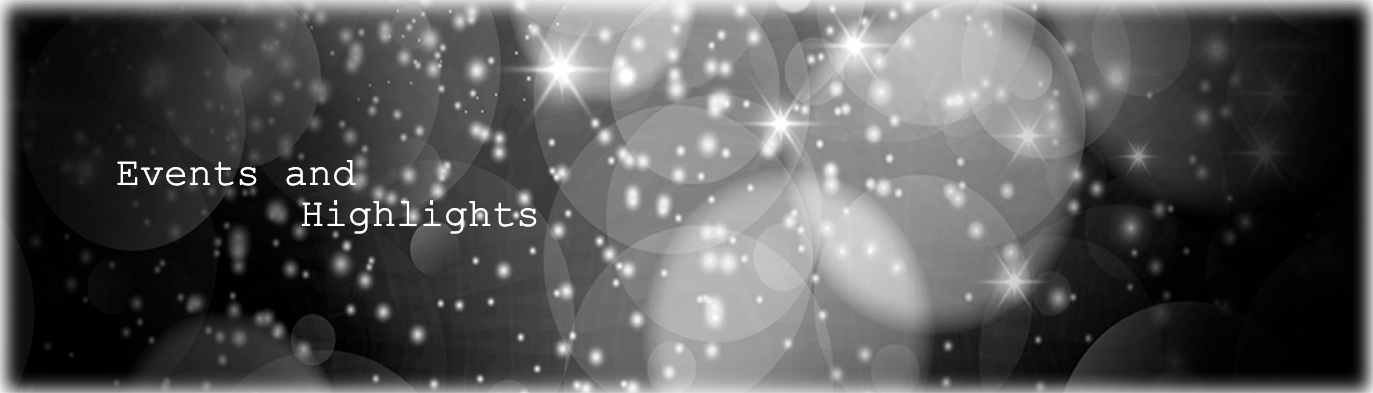
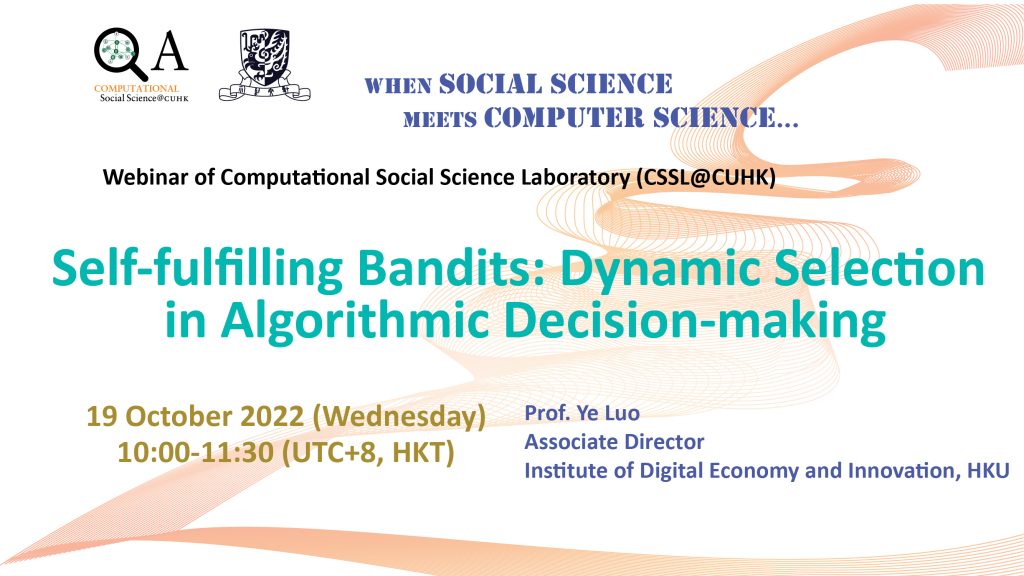
Self-fulfilling Bandits: Dynamic Selection in Algorithmic Decision-making
Abstract:
This talk identifies and addresses dynamic selection problems that arise in online learning algorithms with endogenous data. In a contextual multi-armed bandit model, we show that a novel bias (self-fulfilling bias) arises because the endogeneity of the data influences the choices of decisions, affecting the distribution of future data to be collected and analysed.
A class of algorithms to correct for the bias by incorporating instrumental variables into leading online learning algorithms will be proposed. These algorithms lead to the true parameter values and meanwhile attain low (logarithmic-like) regret levels. I will further prove a central limit theorem for statistical inference of the parameters of interest. To establish the theoretical properties, a general technique that untangles the interdependence between data and actions is developed.
Speaker:
Prof. Ye Luo
Associate Director Institute of Digital Economy and Innovation
University of Hong Kong