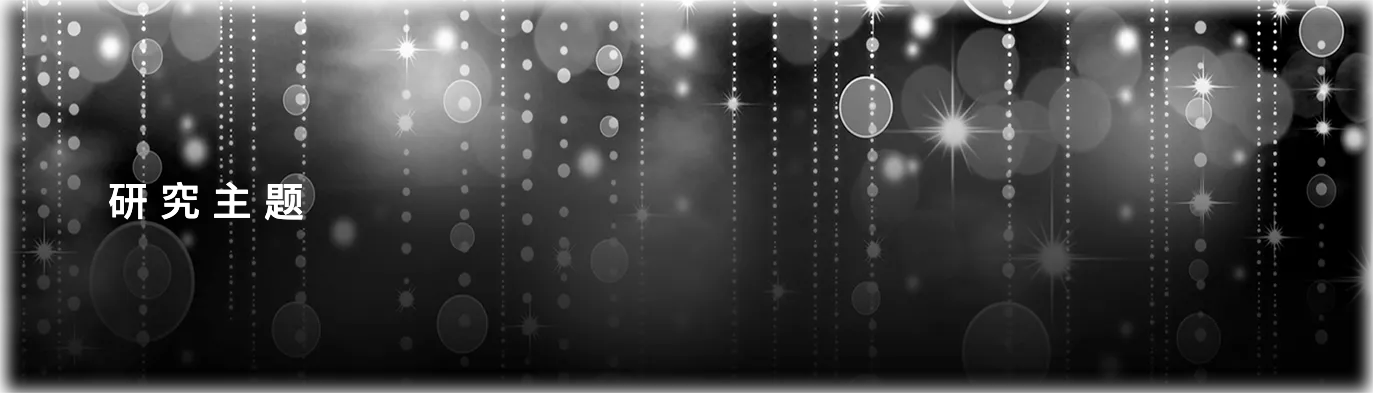
促进社会公益的人工智慧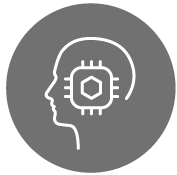
大资料时代极大地改变了经济行为和商业模式,这也给经济研究带来了新的挑战,这些新特征是个人、公司和政策制定者面临的重要问题之一。大量复杂资料和高性能计算能力的日益普及为资料科学家提供了改进现有资料分析模型(或”机器”)和开发新工具以了解资料生成过程机制(从资料中发现因果关系)的机会。这一主题的一个目标是利用新开发的机器学习方法研究重要的经济和金融问题。
该主题的另一个目的是研究各种假设的社会环境和参数的影响下的社会变化动态。主要方法是使用基于代理的类比进行实证研究。不同的假设可以通过计算模型抽象,并通过电脑程式实现。然后,可以分析基于代理的类比实验结果的观察,以便得出结论(例如,社会规范出现的原因、后果和动态)。我们还致力于建立社会动力学的数学模型。这有助于进一步的数学处理,从而产生实验研究中不易获得的结果。
项目:
大维度因果推断和工具变量模型:估计、统计推断和模型选择
(2022-2024)
项目来源:香港研资局General Research Fund(GRF-14617121)
专案统筹者:范青亮教授
In modern policy evaluations, the increasing availability of rich datasets (such as massive administrative data and large survey data) with many individual features (variables) makes the estimation and inference on the heterogeneous or even personalized treatment effect an urgent task for theoretical econometricians and empirical economists. The accurate estimation of heterogeneous treatment effect is important for efficient policy making and social welfare evaluations. The economic policy, e.g., government bailout of small business or individuals during the hardship of COVID-19, would affect people with different economic variables such as debt levels, education, etc., differently. Estimation and inference on the potentially heterogeneous treatment effect is therefore important for modern program evaluations.
This project aims to develop econometric methods to deal with the common issues of causal inference in the form of treatment effect, such as heterogeneity, endogeneity, censored response variable, etc., amid large dimension of control variables and potential instruments. On one hand, more data provides the researchers more information on the subject of their study. On the other hand, it makes the conventional econometric methods infeasible to handle the high-dimensionality nature of the model. Specifically, this research project aims to study the average causal effect using double robustness method that relies on new machine learning methods. We consider the conditional average treatment effect and quantile treatment effect among censored response variables in a high-dimensional model. To address the endogeneity problem we consider a large number of instrumental variables and rely on the variable selection to get the optimal instruments. We also empirically investigate the effect of the firm culture on corporate behavior.
The novelty of our proposed method is that we first investigate the heterogeneous treatment effect with high-dimensional data and complex data structure such as censored response. We innovatively use the dimension reduction technique, taking into account the censored nature of the responses. Then a double robust method is applied to the nonparametric estimation of the heterogeneous treatment effect. Moreover, we consider the both irrelevant and invalid instruments in high-dimensional models.
The results of our study will be of practical use to policy makers, empirical economics researchers and biomedical researchers, etc., providing solutions to the complex data structure when evaluating the heterogeneous treatment effects. We advance the academic literature at the intersection of high-dimensional models, heterogeneous treatment effect, double robust estimation, instrumental variable method, by providing insights on how to make valid inference on the true parameter/functional of interests.
项目:
多智慧体强化学习动力学的形式化建模:平均场理论方法 (2021-2023)
项目来源:香港研资局General Research Fund(GRF 14206820)
专案统筹者:梁浩锋教授
Research into learning through repeated agent interactions in multi-agent systems has become an active topic in the field of multiagent systems. It finds applications in a wide variety of domains. We note that a thorough understanding of multi-agent reinforcement learning is a current topic of research. There has been no research work reported on the formal modelling of the dynamics of multi-agent reinforcement learning for the general
n-agent settings, in particular, when n is very large. However, this is an important research topic. It enables researchers to give a detailed explanation of the process and find out the implications of the process. Generally, there is also a potential that the models can be further developed mathematically. Last but not least, a mathematical model might highlight the importance of certain parameters that would otherwise be overlooked in experimental studies. In this project, we propose to research into the dynamics of such systems with a mean field theoretic approach. We have obtained some very good initial results, which is presented in one of the most prestigious conference in Artificial Intelligence.
There are two specific objectives to be achieved in the project. First, we shall develop a general model of dynamics of multi-agent reinforcement learning in which there are many, many agents. Specifically, a general model will be able to handle the situations that the game of interaction is any game that allow agents to have one-to-one interactions. The model will also be able to allow agents to learn to cooperate with the opponents. The other objective is to build a formal model that incorporates the effects of additional external factors in the process of dynamics of multi-agent reinforcement learning. Examples of such factors are social values, influencer agents, inequity-aversity, and gist traces, to name a few. We hope that the effects of these factors, both on the learning dynamics and on the final outcome, can be analysed theoretically. They will also be verified experimentally if possible.
共同负责人:
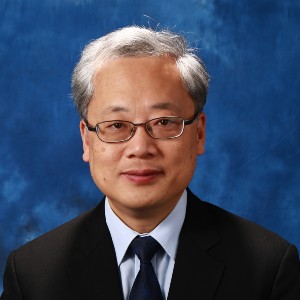
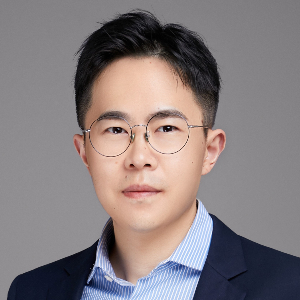